Water Leak Detection
Quantum machine learning solution to optimally position sensors and detect water leaks in urban water systems.
OWNER
STATUS
Phase 2 – Full Proposal
QUANTUM APPROACH
Combinatorial optimisation (quantum inspired)
SDGs
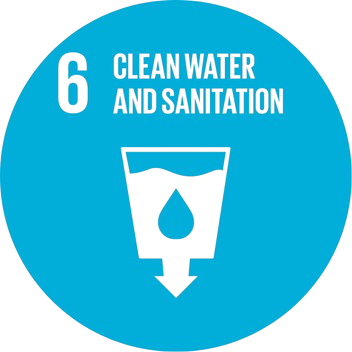
CONTRIBUTORS
QClavis.io
Reply
UNHabitat
ORIGIN OF CONTRIBUTORS
CONTEXT/IMPACT:
Many regions of the world are already water-scarce, and the number of countries affected will increase. The Arab states and many African countries will be severely affected. It is expected that many developed regions in Europe and North America will be faced with critical “day-zero” type scenarios, which have already been experienced in Cape Town and Sao Paulo. Currently Mexico City is precariously close to “day-zero”. The aging infrastructure to collect and distribute water is unable to cope with such demands. By 2050 close to 70% of the world’s population will live in urban space. As a result of increasing populations, many water supply systems are stretched to supply populations beyond their design capacity. Often, such systems have not been properly maintained and have lacked the necessary investment to ensure service levels, especially amongst marginalized groups. From the water resources that are collected and treated, at least half is lost through leakage, system losses or illegal connections. Complex water reticulation systems and pipe networks, particularly those which are old and not maintained, are difficult to monitor. Without a basic level of measurement, interventions to manage the losses are almost impossible.
HOW COULD QUANTUM HELP:
The dynamics of the water distribution network is typically controlled and monitored with a limited number of sensors located at several places in the network. When a leak occurs at some specific location in the network, there is in general a nonlinear signature in the physical quantities (mostly pressure drop) at different locations in the network. From a mathematical standpoint, a given water distribution network can be formulated in the context of graph theory. The main bottleneck to tackle is its combinatorial nature (NP-hard), which limits in general the size of the network that can be efficiently considered. The complexity of this problem makes it a significant focus for research in quantum computing. A promising quantum approach is the use of neutral atom quantum processors, which have a unique advantage in natively embedding complex graph-structured problems like the one relevant for optimal sensor placement at the hardware level, yielding potential advantages in terms of implementation, scalability and performance.