Antimicrobial resistance
Addressing global public health challenges, by developing a quantum computing solution to improve current AI models, predict more quickly and accurately patterns of resistance and identifying new chemical compounds with low resistance on more targeted bacteria.
OWNER
STATUS
Phase 1 – Outline
QUANTUM APPROACH
Machine Learning
SDGs
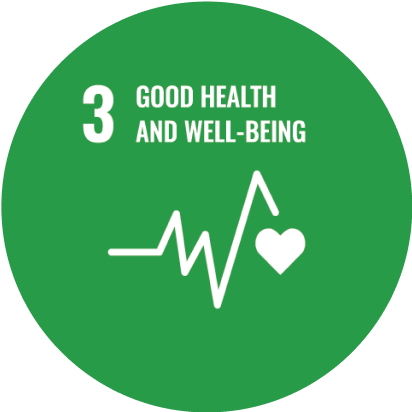
CONTRIBUTORS
University of Copenhagen
Alphanosos
Global Antibiotic Research & Development Partnership (GARDP)
ORIGIN OF CONTRIBUTORS
IMPACT/ CONTEXT
WHO declared antimicrobial resistance (AMR) as one of the top ten threats to global public health.
Low- and middle- income countries, and certain population groups such as children and the elderly, are hit the hardest by AMR. AMR also has an economic cost, as it affects productivity and economic growth. The World Bank estimates that if AMR is unchecked, by 2030, an additional 24.1 million people could be forced into extreme poverty.
Despite the significant economic, environmental, and societal costs, the development of new antimicrobials has not kept pace with the emergence of resistance, leaving healthcare providers with fewer options for treating infections. No new classes of antibiotics have been discovered in the past decades.
HOW QUANTUM COULD HELP
An approach to AMR research is to identify new drugs that have more targeted action to specific diseases and that are efficient against any known resistance mechanism. Machine learning methods are used to find new and efficient combination of compounds. Such predictive models learn relationships between large chemical diversity found in libraries of chemical compounds and experimental in vitro observations, and then predict novel mixes with improved efficacies (in terms of targeted disease treatment and resistance).
A quantum computing solution, based on quantum machine learning, could potentially be developed as an alternative or complement to classical machine learning algorithms for the prediction of patterns of resistance and of new chemical compounds with low resistance on more targeted bacteria. The key goal would be to obtain higher-quality mixes with fewer experimentation-learning cycles, as the number of cycles involves expensive on-demand experiments.