Carbon Capture
Quantum machine learning solution to improve efficiency of catalysts involved in the chemical process of carbon capture.
OWNER
STATUS
Phase 2 – Full Proposal
QUANTUM APPROACH
Machine Learning
SDGs
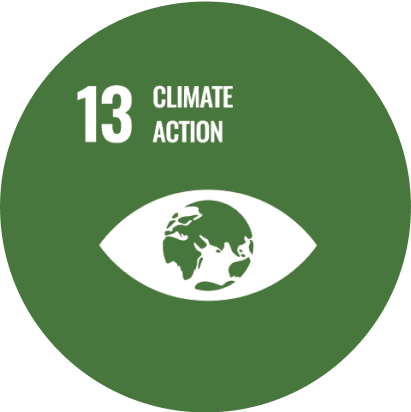
CONTRIBUTORS
QCentroid
ETH Zurich
ORIGIN OF CONTRIBUTORS
CONTEXT/IMPACT:
A primary contributor to climate change is the excessive concentration of carbon dioxide (CO₂) in the atmosphere, mainly from fossil fuel combustion, industrial activities, and deforestation. The rise in CO₂ levels has led to a rapid increase in global temperatures, resulting in extreme weather events, rising sea levels, and disruptions to food and water supplies. Populations in urban areas with dense industrialiaation, such as those in developing countries, face increased health risks from air pollution and climate change-related disasters. Additionally, small island and coastal communities are disproportionately affected by rising sea levels and extreme weather events. The Paris Agreement, adopted under the United Nations Framework Convention on Climate Change (UNFCCC), aims to limit the global temperature increase to well below 2°C, with efforts to keep it to 1.5°C above pre-industrial levels. Achieving these goals requires significantly reducing greenhouse gas emissions, of which carbon capture technologies play a critical role. Catalytic reactions inherent to the carbon capture process yet need to improve its energy-efficiency. Moreover, Carbon Capture, Utilization, and Storage (CCUS) technologies not only reduce the overall carbon footprint but also contribute to the development of a circular carbon economy, where CO₂ is seen as a valuable resource rather than waste. Metal-organic frameworks (MOFs) have emerged as promising materials for Direct Air Capture (DAC) due to their tunable structures. However, identifying and optimizing MOFs involves navigating a vast space of possible configurations, which is a complex and resource-intensive task.
HOW COULD QUANTUM HELP:
A major challenge in developing sustainable catalytic processes lies in accurately modeling molecular interactions at the atomic level. Existing approaches are often constrained by the approximations inherent in classical models, which can lead to potential inaccuracies in predicting molecular behaviors. Additionally, the computational power required to model large and complex systems is immense, which can be prohibitive and slow down the pace of discovery and innovation. Identifying and optimising MOFs involves navigating a vast space of possible configurations. This is a complex and resource-intensive task, which can be done with Generative Adversarial Networks (GANs). A main limitation with classical GANs is the training instabilities that require a substantial amount of computational resources to model such large spaces accurately. Quantum computing, particularly through Quantum Generative Adversarial Networks (QGANs), could offer a promising alternative. Instead of relying solely on classical calculations, QGANs could be employed to generate new chemical catalysts and MOFs more efficiently by making use of quantum noise for the generative network, enhancing the ability to explore vast chemical spaces, identify novel materials and catalytic processes.